Visual representation of a decision tree
In this example we are going to plot a decision tree of type DecisionTree.jl
using the Bucheim Layout from NetworkLayout.jl
.
using CairoMakie
using Graphs
using GraphMakie
import MLJ
using NetworkLayout
using DecisionTree
This following code, which walks the tree and creates a SimpleDiGraph
was taken and slightly modified from syntaxtree.jl
. Thanks!
The model is a DecisionTree object. maxdepth defines the max Depth of the final tree generated.
import Base.convert
function Base.convert(::Type{SimpleDiGraph},model::DecisionTree.DecisionTreeClassifier; maxdepth=depth(model))
if maxdepth == -1
maxdepth = depth(model)
end
g = SimpleDiGraph()
properties = Any[]
walk_tree!(model.root.node,g,maxdepth,properties)
return g, properties
end
function walk_tree!(node::DecisionTree.Node, g, depthLeft, properties)
add_vertex!(g)
if depthLeft == 0
push!(properties,(Nothing,"..."))
return vertices(g)[end]
else
depthLeft -= 1
end
current_vertex = vertices(g)[end]
val = node.featval
featval = isa(val,AbstractString) ? val : round(val;sigdigits=2)
push!(properties,(Node,"Feature $(node.featid) < $featval ?"))
child = walk_tree!(node.left,g,depthLeft,properties)
add_edge!(g,current_vertex,child)
child = walk_tree!(node.right,g,depthLeft,properties)
add_edge!(g,current_vertex,child)
return current_vertex
end
function walk_tree!(leaf::DecisionTree.Leaf, g, depthLeft, properties)
add_vertex!(g)
n_matches = count(leaf.values .== leaf.majority)
#ratio = string(n_matches, "/", length(leaf.values))
push!(properties,(Leaf,"$(leaf.majority)"))# : $(ratio)"))
return vertices(g)[end]
end
Ooof, quite a bit of code!
Makie @recipe
Now let's define a MakieRecipe for the plot, to make plotting easy
@recipe(PlotDecisionTree) do scene
Attributes(
nodecolormap = :darktest,
textcolor = RGBf(0.5,0.5,0.5),
leafcolor = :darkgreen,
nodecolor = :white,
maxdepth = -1,
)
end
import GraphMakie.graphplot
import Makie.plot!
function GraphMakie.graphplot(model::DecisionTreeClassifier;kwargs...)
f,ax,h = plotdecisiontree(model;kwargs...)
hidedecorations!(ax); hidespines!(ax)
return f
end
function plot!(plt::PlotDecisionTree{<:Tuple{DecisionTreeClassifier}})
@extract plt leafcolor,textcolor,nodecolormap,nodecolor,maxdepth
model = plt[1]
# convert to graph
tmpObs = @lift convert(SimpleDiGraph,$model;maxdepth=$maxdepth)
graph = @lift $tmpObs[1]
properties = @lift $tmpObs[2]
# extract labels
labels = @lift [string(p[2]) for p in $properties]
# set the colors, first for nodes & cutoff-nodes, then for leaves
nlabels_color = map(properties, labels, leafcolor,textcolor,nodecolormap) do properties,labels,leafcolor,textcolor,nodecolormap
# set colors for the individual elements
leaf_ix = findall([p[1] == Leaf for p in properties])
leafValues = [p[1] for p in split.(labels[leaf_ix]," : ")]
# one color per category
uniqueLeafValues = unique(leafValues)
individual_leaf_colors = resample_cmap(nodecolormap,length(uniqueLeafValues))
nlabels_color = Any[p[1] == Node ? textcolor : leafcolor for p in properties]
for (ix,uLV) = enumerate(uniqueLeafValues)
ixV = leafValues .== uLV
nlabels_color[leaf_ix[ixV]] .= individual_leaf_colors[ix]
end
return nlabels_color
end
# plot :)
graphplot!(plt,graph;layout=Buchheim(),
nlabels=labels,
node_size = 100,
node_color=nodecolor,
nlabels_color=nlabels_color,
nlabels_align=(:center,:center),
##tangents=((0,-1),(0,-1))
)
return plt
end
Visualizing a DecisionTree
Now finally we are ready to fit & visualize the tree
iris = MLJ.load_iris()
features = hcat(iris[1],iris[2],iris[3],iris[4])
labels = iris[5]
model = DecisionTreeClassifier(max_depth=4)
fit!(model, features, labels)
DecisionTreeClassifier
max_depth: 4
min_samples_leaf: 1
min_samples_split: 2
min_purity_increase: 0.0
pruning_purity_threshold: 1.0
n_subfeatures: 0
classes: ["setosa", "versicolor", "virginica"]
root: Decision Tree
Leaves: 8
Depth: 4
now we are ready to plot
graphplot(model)

you can also specify depth, or modify colors
graphplot(model;maxdepth=3,textcolor=:darkgreen)
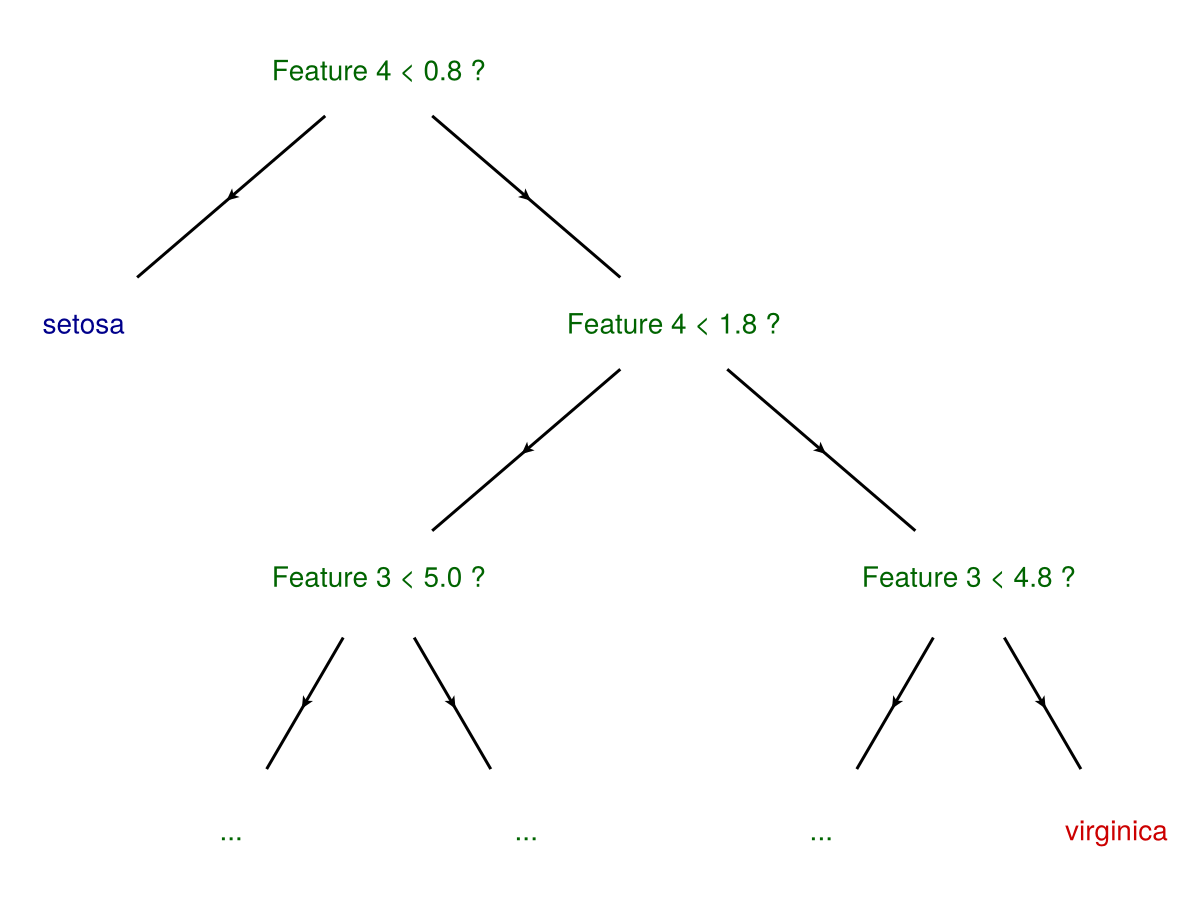
This page was generated using Literate.jl.